Leveraging Image Datasets for Superior Object Detection
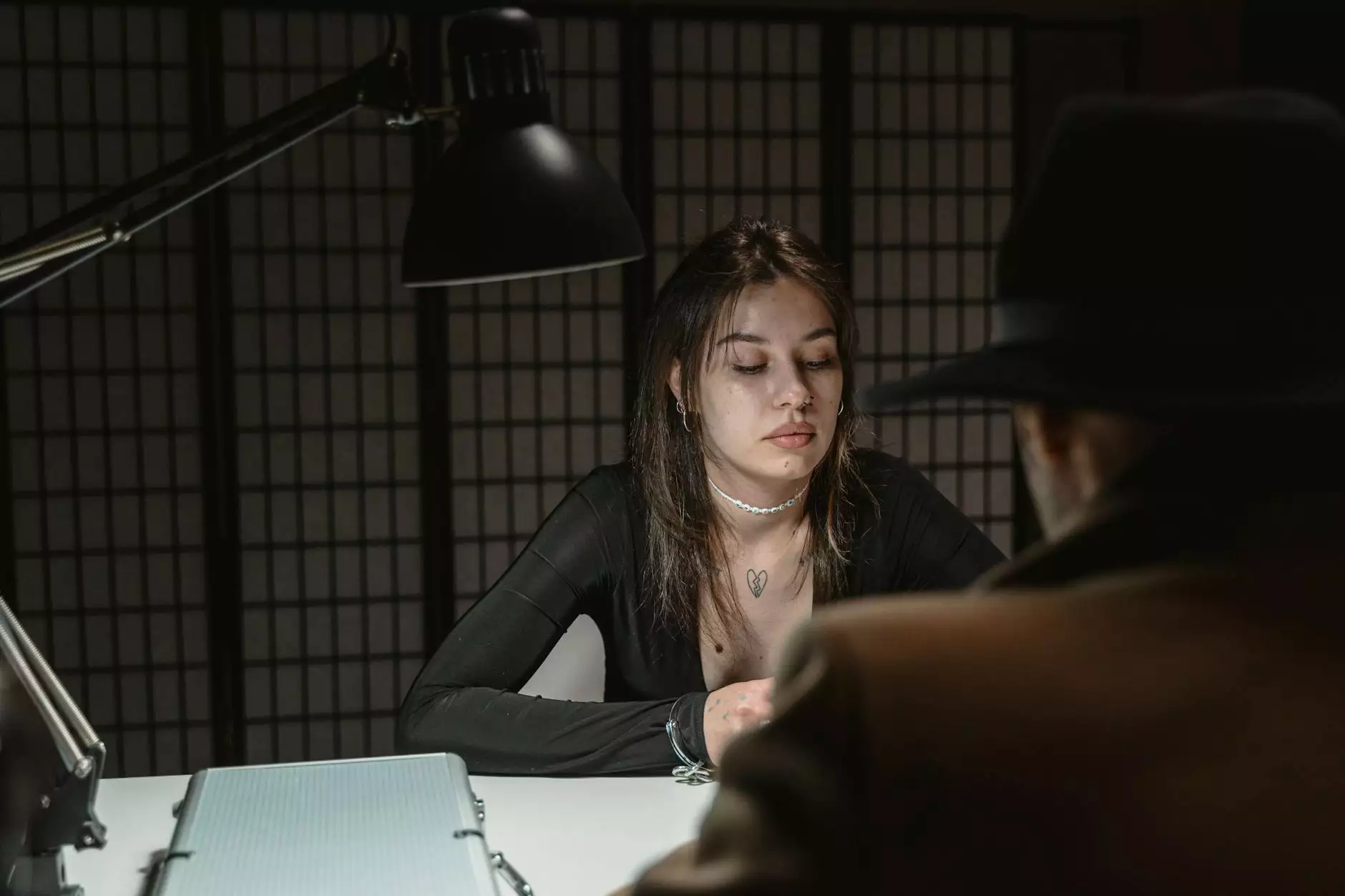
In today’s technology-driven world, businesses are increasingly turning to image datasets for object detection to enhance their visual data processing capabilities. Whether it’s for developing cutting-edge computer vision applications or improving operational efficiency, understanding how to effectively leverage image datasets can set your business apart from the competition.
The Importance of Image Datasets in Object Detection
Object detection is a pivotal aspect of many applications within artificial intelligence and machine learning. The foundation of any successful object detection system lies in the quality and comprehensiveness of the image datasets used for training. Below are several reasons why image datasets are vital for object detection:
- High Accuracy: Quality datasets lead to high accuracy in identifying and classifying objects.
- Diverse Data: Comprehensive datasets ensure models can generalize well to real-world scenarios.
- Reduced Overfitting: Well-curated datasets can help mitigate the risk of overfitting, making models robust.
- Better Training: Large and varied datasets allow for more effective training of machine learning algorithms.
What Constitutes a Quality Image Dataset?
A quality image dataset for object detection needs to embody several critical characteristics:
- Annotated Images: Each image in the dataset should have accurate annotations that reflect the objects present.
- Diversity in Images: The dataset must contain a wide variety of images, illustrating various conditions such as lighting, angles, and backgrounds.
- Volume: A substantial number of images enhance the model's learning capabilities and accuracy.
- Realism: The images should represent real-world scenarios to ensure that the trained model performs effectively in practical settings.
Data Annotation Tools: Essential for Creating Image Datasets
Data annotation tools play a crucial role in the preparation of image datasets for object detection. These tools allow businesses to efficiently annotate and curate large volumes of image data. Some of the key features to look for in a data annotation tool include:
1. User-Friendly Interface
It’s essential for data annotation tools to have an intuitive interface, facilitating quick learning and reducing the time required for training annotators.
2. Support for Various Annotation Types
Support for different types of annotations—including bounding boxes, polygon segmentation, and image classification—is critical for comprehensive object detection.
3. Collaborative Features
With multiple teams often working on the same dataset, collaborative features can streamline the annotation process and improve communication.
4. Integration Capabilities
Data annotation tools should seamlessly integrate with existing machine learning pipelines and platforms to ensure a smooth workflow.
KeyLabs.ai: Your Partner in Data Annotation
At KeyLabs.ai, we offer a state-of-the-art data annotation platform designed to simplify the process of creating high-quality image datasets for object detection. Our platform combines cutting-edge technology with a user-friendly design, ensuring that your annotation projects run smoothly and efficiently.
Our Features Include:
- Automated Annotation: Reduces manual effort, speeds up the annotation process.
- Quality Control: Layers of review and quality checks to maintain high standards.
- Real-Time Collaboration: Teams can work together from anywhere in the world, enhancing productivity.
- Custom Annotation Tools: Tailored to meet your unique project needs.
Best Practices for Building Effective Image Datasets
Creating an effective image dataset is an intricate process that requires strategic planning and execution. Here are some best practices:
1. Define Your Objectives
Be clear about what you want your object detection model to achieve. This helps in designing your dataset accordingly.
2. Collect Diverse Data
Diversity in your dataset will improve the model’s learning capacity. Aim to collect images from various sources and environments.
3. Ensure High-Quality Images
Low-quality images can lead to poor model performance. Ensure only high-resolution images are included in the dataset.
4. Annotate with Precision
Inaccurate annotations can drastically impact the accuracy of the model. Employ skilled annotators and robust quality control measures.
The Future of Object Detection: Trends to Watch
The landscape of object detection is continuously evolving. Here are some trends to keep an eye on as you navigate the future of image datasets:
1. Advancements in Deep Learning
Deep learning techniques are becoming more sophisticated, allowing for improved accuracy and performance in object detection.
2. Synthetic Data Generation
With the challenges of gathering real-world data, synthetic datasets are gaining traction, offering varied scenarios for training models.
3. Increased Use of AI in Annotation
AI-driven annotation tools are becoming more prevalent, automating parts of the annotation process and increasing efficiency.
4. Ethical and Inclusive AI
The focus on creating inclusive datasets that avoid bias will be paramount as the industry moves forward.
Conclusion: Transforming Your Business with Image Datasets
In conclusion, the strategic use of image datasets for object detection can transform your business operations, leading to enhanced decision-making, improved customer experiences, and superior operational efficiencies. By embracing quality data annotation tools and platforms such as KeyLabs.ai, your organization can leverage the full power of object detection technology. As you invest in these resources, you're not just improving your models; you're paving the way for innovative solutions that can reshape your industry.
Always remember, the quality of your data influences the success of your AI applications. By focusing on building robust image datasets, you are setting the groundwork for meaningful advancements in object detection and beyond.